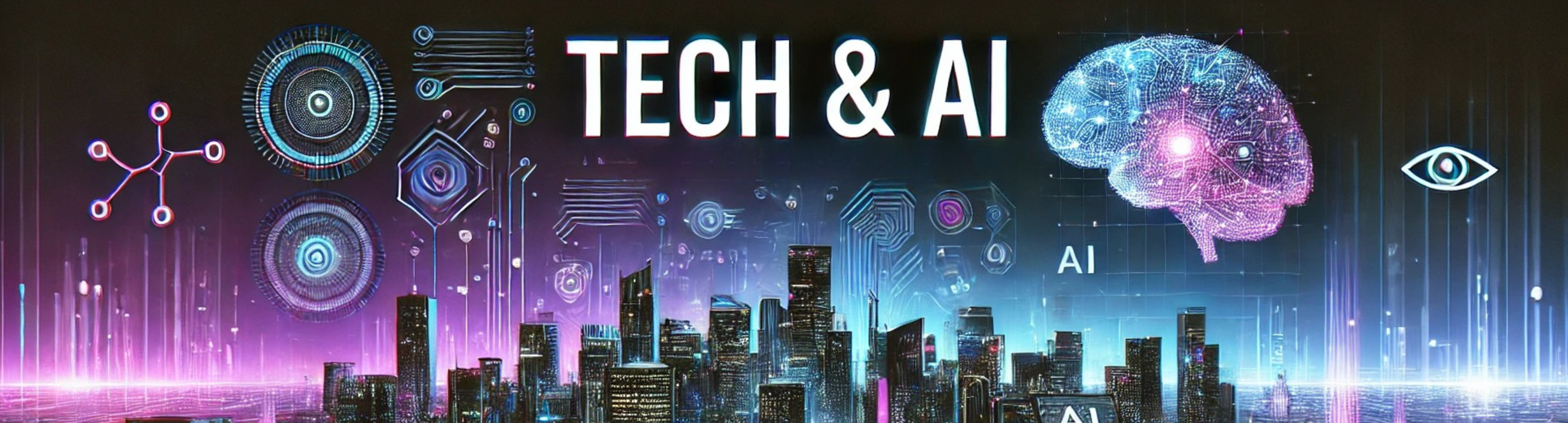
Tech and AI: The Future of Humanity
In the 21st century, technology and artificial intelligence (AI) have become inseparable from our daily lives. From the smartphones we carry in our pockets to the algorithms that curate our social media feeds, AI is quietly revolutionizing the way we live, work, and interact with the world. The rapid advancements in technology and AI are not just transforming industries; they are reshaping the very fabric of human society. This blog delves into the intricate relationship between technology and AI, exploring their history, current applications, ethical implications, and the future they are shaping.
The Evolution of Technology and AI
To understand the profound impact of AI, it is essential to trace the evolution of technology and AI. The journey of technology dates back to the invention of the wheel, the printing press, and the steam engine—each of which marked a significant leap in human progress. However, the advent of computers in the mid-20th century marked the beginning of a new era. The development of the first programmable computer, ENIAC, in 1945, laid the foundation for modern computing.
The concept of AI, however, emerged much later. The term “artificial intelligence” was coined in 1956 by John McCarthy during the Dartmouth Conference, where a group of scientists gathered to explore the possibility of creating machines that could mimic human intelligence. Early AI research focused on symbolic AI, which involved creating rules and logic-based systems to solve problems. However, progress was slow due to limited computational power and data.
The 21st century witnessed a paradigm shift in AI with the advent of machine learning (ML) and deep learning (DL). Machine learning algorithms, which enable computers to learn from data, became the cornerstone of modern AI. The availability of massive datasets, coupled with advancements in hardware such as GPUs, allowed researchers to train complex neural networks. This led to breakthroughs in areas such as image recognition, natural language processing (NLP), and autonomous systems.
The Current Landscape of AI
Artificial Intelligence (AI) has evolved from a theoretical concept into a transformative force reshaping industries, economies, and daily life. From automation in businesses to advancements in healthcare, AI is at the forefront of technological innovation. The rapid growth in AI capabilities, largely driven by machine learning (ML) and deep learning (DL), has resulted in applications that were once thought to be purely within the realm of science fiction. In this blog, we will explore the current landscape of AI, its impact across various domains, the ethical and regulatory challenges, and future trends that will define its trajectory.
1. Healthcare
AI is revolutionizing healthcare by enabling early diagnosis, personalized treatment, and drug discovery. Machine learning algorithms can analyze medical images, such as X-rays and MRIs, to detect diseases like cancer with remarkable accuracy. AI-powered tools are also being used to predict patient outcomes, optimize hospital operations, and even assist in surgeries. For instance, robotic surgery systems, such as the Da Vinci Surgical System, use AI to enhance precision and reduce the risk of complications.
2. Finance
The financial industry has embraced AI to improve decision-making, detect fraud, and enhance customer experiences. AI algorithms analyze vast amounts of financial data to identify patterns and make predictions. For example, robo-advisors use AI to provide personalized investment advice, while fraud detection systems use machine learning to identify suspicious transactions in real-time. AI is also transforming trading, with algorithmic trading systems executing trades at lightning speed based on market data.
AI Scope and Challenges
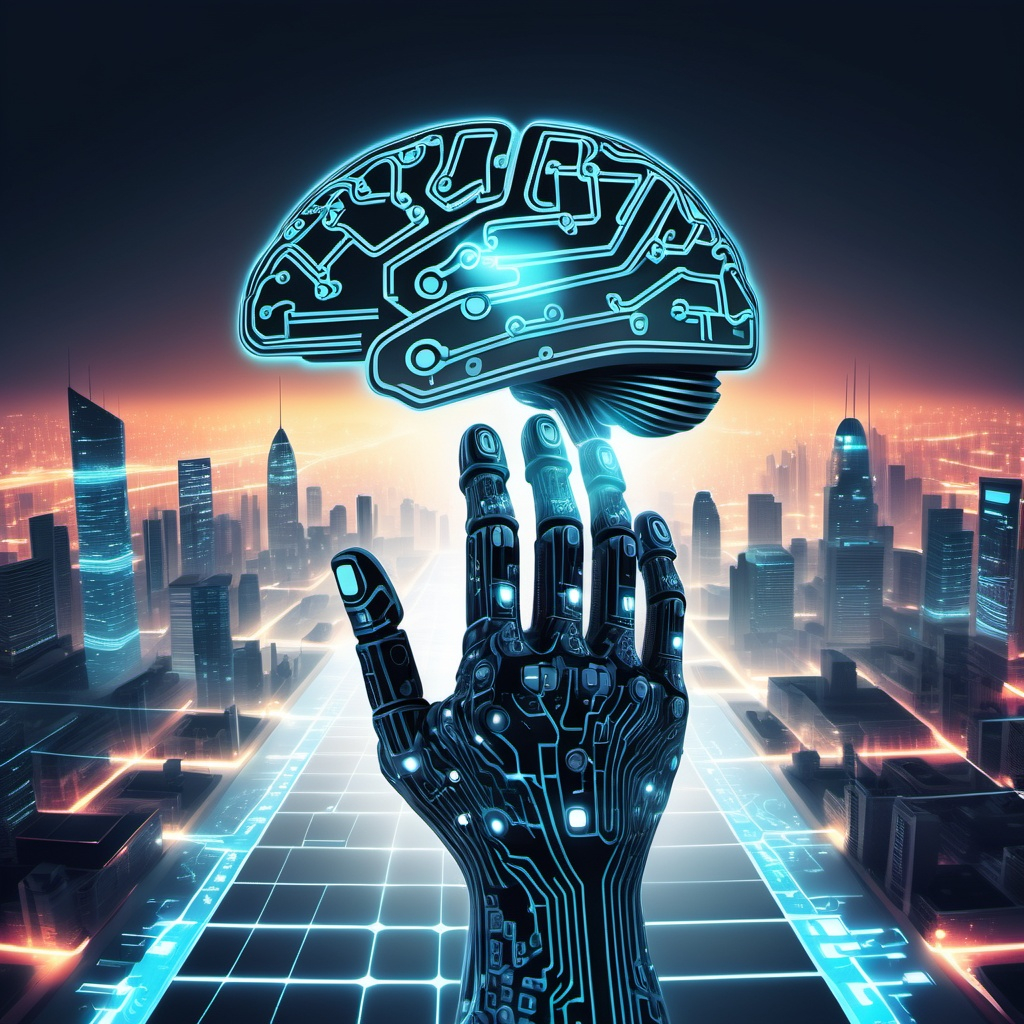
AI has significantly impacted multiple industries, including healthcare, finance, retail, manufacturing, education, transportation, and cybersecurity. In healthcare, AI-powered tools analyze medical images with high accuracy, while financial institutions use AI for fraud detection and risk assessment. AI enhances customer experiences in retail, enables predictive maintenance in manufacturing, and contributes to autonomous vehicles in transportation. It also plays a crucial role in cybersecurity by identifying and neutralizing threats in real time.
However, AI development and deployment come with challenges. Data privacy and security remain major concerns as AI systems rely heavily on vast amounts of data. Bias in AI models can lead to unfair outcomes, necessitating ethical AI development. Regulatory and legal frameworks are evolving to ensure responsible AI usage, balancing innovation and compliance. Additionally, AI systems require immense computational power, raising sustainability concerns.
A Comprehensive Exploration of AI's Evolution, Applications, and Challenges
1. The Early Foundations of AI
AI’s roots trace back to the mid-20th century, when researchers began to speculate about the possibility of creating machines capable of simulating human intelligence. Early pioneers like Alan Turing posed fundamental questions, such as whether machines could ever truly “think.” While computing power and algorithmic sophistication were limited at the time, these foundational ideas laid the groundwork for what would eventually become a seismic shift in technology.
During the 1950s and 1960s, significant strides were made in symbolic AI—where systems attempted to replicate logical reasoning using rules and knowledge bases. Expert systems, which emerged in the 1970s, aimed to encapsulate human expertise in codified sets of if-then statements. Although these systems were limited by the computational constraints of the era and the brittleness of hardcoded rules, they showcased the potential of AI to solve specialized tasks. Notably, these early achievements foreshadowed contemporary AI’s broad applicability, even if the true explosion of AI capabilities would not occur until decades later when hardware and data availability caught up with theoretical ambitions.
2. The Rise of Machine Learning
The modern age of AI began to crystallize around the mid-2000s with the advent of accessible large-scale data sets—often referred to as “Big Data.” This avalanche of data became the lifeblood for machine learning algorithms. Supervised, unsupervised, and reinforcement learning techniques began to mature, allowing computers to learn patterns and relationships from data rather than relying on meticulously crafted rules. Techniques like decision trees, support vector machines, and ensemble methods like Random Forests found widespread use across industries. Enterprises discovered they could gain competitive advantages by automating tasks such as credit scoring, inventory management, and targeted marketing.
However, it was the renaissance of neural networks—especially deep learning—that propelled AI into the mainstream in the 2010s. Pioneering researchers like Geoffrey Hinton, Yann LeCun, and Yoshua Bengio demonstrated that multi-layer neural networks, given sufficient computational power (facilitated by GPUs) and vast labeled datasets, could exceed previous AI methods in tasks such as image classification and speech recognition. Convolutional Neural Networks (CNNs) unlocked breakthroughs in computer vision, Recurrent Neural Networks (RNNs) revolutionized language-related tasks, and more advanced architectures like LSTM (Long Short-Term Memory) and Transformer-based models opened up new frontiers in text and sequence processing.
This deep learning revolution led to superhuman performance in tasks that had long eluded AI, such as Go, poker, and various complex video games. Companies swiftly integrated deep learning into products for image search, voice assistants, translation, and much more, fueling a surge in AI research and development across the globe.
3. Natural Language Processing (NLP) and the Advent of Large Language Models
One of AI’s most mesmerizing achievements in recent years lies in the field of Natural Language Processing (NLP). Early NLP efforts struggled to grasp the nuances of language—its grammar, context, and semantic richness. Rule-based systems and simpler statistical models like n-grams offered limited capabilities, often failing to capture context beyond short windows.
The introduction of word embeddings such as Word2Vec, GloVe, and later the Transformer architecture revolutionized how machines process language. Transformers, which rely on attention mechanisms, enabled models to handle long-range dependencies in text more effectively. The release of models like BERT (Bidirectional Encoder Representations from Transformers) demonstrated that by pre-training on vast corpora, systems could learn deeply contextual embeddings and then be fine-tuned for a range of NLP tasks—question answering, sentiment analysis, and named entity recognition—with remarkable results.
As hardware capabilities soared, the field saw the emergence of large language models (LLMs). GPT (Generative Pre-trained Transformer) series from OpenAI, T5 from Google, and other massive architectures illustrated that with billions or even trillions of parameters, language models could exhibit a surprising level of fluency, creativity, and adaptability. This breakthrough sparked debates regarding AI’s role in content generation, the future of creative industries, the potential for misinformation, and the ethical boundaries of machine-generated narratives.
4. Computer Vision, Image Recognition, and Beyond
Alongside NLP, computer vision has been a primary beneficiary of deep learning. ImageNet, a massive labeled dataset containing millions of images across numerous categories, served as a pivotal benchmark. In 2012, a deep convolutional neural network called AlexNet stunned the world by shattering previous records in image classification, igniting the “ImageNet moment” that heralded deep learning’s broader adoption.
Subsequent enhancements in CNN architectures (VGG, Inception, ResNet, EfficientNet, etc.) have enabled AI systems to achieve superhuman accuracy in tasks such as image recognition, object detection, and semantic segmentation. Beyond static images, computer vision has expanded to include video analysis, action recognition, and 3D scene understanding, enabling AI-driven video surveillance, advanced driver-assistance systems, and even real-time sports analytics.
One of the most transformative applications of computer vision is in the healthcare domain, where AI-driven medical imaging assists radiologists in detecting diseases such as cancer or identifying early signs of conditions like diabetic retinopathy. By automating these time-intensive diagnostic tasks or providing second opinions, AI has significantly improved clinical outcomes and efficiency. Yet, questions regarding accountability, data privacy, and the necessity for clinical validation remain central to discussions about the responsible integration of AI into healthcare.
5. Robotics and the Edge of Autonomy
For many, the ultimate manifestation of AI is the robot—an embodied machine that perceives the world, makes decisions, and takes actions. Modern AI-powered robotics has advanced well beyond simple industrial arms executing repetitive tasks. The fusion of computer vision, motion planning, reinforcement learning, and sensor fusion technologies now allows robots to adapt in real time to changing environments.
In warehouses, robots navigate independently, picking and sorting items. On factory floors, collaborative robots (cobots) safely work alongside human operators. Outside controlled settings, drones for delivery and self-driving vehicles exemplify the potential (and complexity) of AI in real-world autonomy. Tesla’s Autopilot, Waymo’s self-driving taxis, and countless research initiatives underscore the unprecedented technical and ethical challenges in handing over control to AI in scenarios that involve human lives and dynamic environments.
Despite high-profile setbacks and controversies, the trend toward increased autonomy in everything from personal drones to public transport systems remains strong. The promise of reduced accidents, improved logistical efficiencies, and more inclusive mobility solutions drives significant investment in these technologies.
6. AI in Healthcare, Finance, and Other Major Industries
Beyond robotics, AI’s imprint is visible across every major industry:
Healthcare: AI-driven diagnostic tools, personalized medicine, patient data management, and public health analytics.
Finance: High-frequency trading, automated risk assessment, fraud detection, algorithmic investing, and AI-driven customer service.
Retail: Personalized recommendations, supply chain optimization, inventory forecasting, customer analytics, and automated checkout systems.
Manufacturing: Predictive maintenance, quality control through computer vision, robotic automation, digital twins, and real-time supply chain oversight.
Education: Adaptive learning platforms, automated grading, language learning apps, tutoring systems, and predictive student performance analytics.
Cybersecurity: Advanced threat detection, behavioral analytics, anomaly detection, and AI-assisted security operations centers.
Agriculture: Precision farming, yield optimization, disease detection in crops, drone surveillance, and supply chain automation.
Entertainment: Personalized content recommendations, virtual characters, deepfake technology, and interactive gaming experiences.
The breadth of AI’s integration signals a future in which industries are ever more data-driven and algorithmically optimized. However, such pervasive adoption also underscores the complexity of AI’s societal impact, from labor market disruptions to shifts in consumer behaviors.
7. Ethical Considerations: Bias, Fairness, and Accountability
With AI’s growing authority in decision-making processes, questions of bias and fairness have taken center stage. Machine learning models are trained on data that may reflect historical inequalities or cultural stereotypes. Consequently, an AI system could unintentionally discriminate in hiring, lending, or even medical treatment recommendations if its training data is skewed. These issues have prompted calls for better data collection practices, more diverse development teams, algorithmic audits, and frameworks that promote fairness, accountability, and transparency.
Models like facial recognition systems have already demonstrated racial and gender biases in multiple studies, leading to misidentification or higher error rates for minorities. The public outcry over such findings has spurred debates on regulatory oversight, calls to ban or restrict certain AI applications, and demands for more robust internal governance within tech companies.
Moreover, the concept of “explainable AI” has gained traction. Stakeholders—ranging from healthcare patients to financial customers—want clarity on how AI systems arrive at decisions that can significantly affect their lives. Interpretable models, feature attribution methods, and post-hoc explanations are actively being researched and developed to satisfy the desire for clarity without sacrificing too much accuracy.
8. Data Privacy and Security
Data is the lifeblood of AI. Yet, the massive collection of personal data—ranging from browsing habits and social media activity to biometric data in healthcare—has generated intense scrutiny over privacy. Regulations like the European Union’s General Data Protection Regulation (GDPR) and the California Consumer Privacy Act (CCPA) aim to give individuals more control over their personal data and impose stricter obligations on organizations that handle it.
While these regulations are steps toward greater accountability, they also illustrate a tension: AI thrives on large, comprehensive datasets, but privacy laws can constrain data collection and sharing. Privacy-preserving techniques like federated learning, differential privacy, and secure multi-party computation have emerged as potential solutions that allow model training without direct data exchange. Still, these technologies remain in relatively early stages, and implementing them at scale can be challenging.
Cybersecurity is another dimension that intersects with AI. On one hand, AI can detect intrusions and malware more effectively by analyzing network behaviors and identifying anomalies. On the other hand, AI systems themselves become targets of sophisticated attacks, ranging from data poisoning (manipulating training data to undermine model performance) to adversarial inputs crafted to fool AI into misclassification.
9. The Regulatory Landscape
Governments worldwide grapple with how best to regulate AI, balancing the desire for innovation with public safety and ethical norms. Some jurisdictions have taken proactive stances:
The EU has proposed comprehensive AI regulations that categorize AI applications based on risk, imposing stricter obligations on higher-risk systems. This approach seeks to govern facial recognition, critical infrastructure, and other sensitive domains.
The U.S. has taken a more fragmented approach, with sector-specific regulations, while fostering innovation through initiatives like the National AI Initiative Office.
China, intent on becoming a global AI leader, invests heavily in AI research while also deploying AI for state surveillance, sparking debates over digital authoritarianism and the global export of AI-based surveillance technologies.
These differing regulatory philosophies underscore the challenges of creating globally consistent AI standards. Moreover, the rapid evolution of AI often outstrips the slower processes of lawmaking. Industry-wide self-governance, ethical guidelines from organizations like the Institute of Electrical and Electronics Engineers (IEEE), and ongoing academic discourse all play critical roles in shaping the ethical deployment of AI.
10. AI and the Future of Work
Automation through AI, robotics, and other advanced technologies raises questions about employment. Will AI displace human workers en masse, or will it create new categories of jobs? Historical technological revolutions—like the Industrial Revolution—have led to shifts in labor markets but ultimately created new opportunities. AI’s impact may be more profound and rapid, potentially requiring major societal adaptations, re-skilling programs, and policy interventions like universal basic income or job transition initiatives.
Jobs that involve repetitive tasks—be they manual or cognitive—are most vulnerable to automation. However, roles requiring creativity, critical thinking, interpersonal skills, and complex decision-making are less likely to be replaced outright. Many experts argue that AI will augment human capabilities, turning humans into “super-workers” who can leverage AI to handle mundane tasks, improve decision-making, and expand productivity.
11. AI for Social Good
Not all AI applications are profit-driven. Non-governmental organizations, social enterprises, and humanitarian agencies increasingly use AI to tackle issues like poverty, climate change, healthcare accessibility, and disaster response. For instance, machine learning models can predict areas at risk of food shortages, enabling timely aid distribution. Computer vision-based drones can survey disaster-stricken areas to guide rescue efforts. AI-driven climate models help researchers understand complex environmental systems and inform policy.
While these initiatives showcase AI’s potential for social impact, they also highlight the resource and knowledge disparities across the globe. Many developing regions have limited access to AI research, infrastructure, and educational resources. Bridging this digital divide will be crucial to ensuring equitable benefits from AI technologies.
12. The Next Frontier: Generative AI
One of the most captivating developments in the last few years is Generative AI. Systems like GANs (Generative Adversarial Networks) and diffusion models can create remarkably realistic images, videos, and audio, while large language models can generate human-like text. Use cases range from entertainment (creating lifelike virtual actors) to design and prototyping (rapidly generating design ideas or synthetic data). Yet, generative AI also spawns deepfakes—fabricated media that can be used maliciously to spread disinformation or commit fraud.
The potential for generative AI is immense, from helping architects visualize new structures to allowing filmmakers to recreate historical scenes seamlessly. It also adds complexity to ethical and social considerations, especially when discerning real from fabricated content becomes increasingly difficult.
13. Reinforcement Learning and Decision-Making
Reinforcement Learning (RL) algorithms, which learn by receiving rewards for certain actions, have been particularly successful in game-based scenarios. AlphaGo, AlphaZero, and MuZero from DeepMind exemplify how RL can master complex board and video games. Beyond gaming, RL is applied in robotics for tasks like grasping and balancing, and in recommendation systems to optimize long-term user engagement.
However, deploying RL in real-world environments requires careful risk management. Poorly designed reward functions can incentivize undesirable behaviors, and the trial-and-error nature of RL is impractical or unsafe in contexts where errors carry high stakes—like healthcare or finance.
14. AI Hardware and Infrastructure
AI breakthroughs are not just about algorithms—they also depend on specialized hardware and large-scale infrastructure. GPUs from companies like NVIDIA initially fueled the deep learning boom, and newer chip architectures (TPUs, FPGAs, custom AI accelerators) continue to push performance boundaries. Cloud providers offer specialized AI services, democratizing access to powerful hardware and software stacks. Edge computing is another emerging domain, enabling AI processing on-device rather than relying heavily on remote servers.
As AI models grow in size and complexity, resource requirements skyrocket, raising concerns over energy consumption and environmental impact. Data centers require massive amounts of electricity to operate and cool. Efforts to build more efficient models, utilize green energy, and design more power-efficient hardware are ongoing, but striking a balance between AI advancement and sustainability remains a significant challenge.
15. Societal and Philosophical Implications
Beyond technical and commercial considerations, AI provokes deeper philosophical questions. If AI were to reach or surpass human-level intelligence—often termed Artificial General Intelligence (AGI)—how would that reshape human society, labor, governance, and even our conception of consciousness and self?
While current AI remains narrow—exceling at specific tasks rather than general intelligence—the possibility of AGI continues to drive debate. Tech luminaries like Ray Kurzweil predict a “singularity” where machine intelligence grows exponentially, while others are more skeptical, pointing to fundamental barriers in replicating human cognition. Regardless of the timeline, the notion that machines could one day rival human intelligence prompts policymakers, ethicists, and researchers to contemplate existential risks, moral responsibilities, and strategies for safe AI alignment.
16. AI in a Post-Pandemic World
The COVID-19 pandemic accelerated the adoption of digital technologies. Remote work became the norm, e-commerce flourished, and telemedicine gained widespread acceptance. AI played a key role in vaccine development (through computational biology), contact tracing, and analyzing massive troves of pandemic-related data.
As the world recalibrates, AI will likely remain integral in shaping more resilient supply chains, healthcare systems, and remote collaboration tools. The pandemic provided a proof of concept for how rapidly societies can pivot with the aid of digital and AI-driven solutions, but it also highlighted issues of data privacy and the digital divide. Organizations are now more conscious of building flexible, AI-centric infrastructures that can respond rapidly to future disruptions.
17. Collaboration vs. Competition in AI Research
AI research flourishes in global ecosystems, with academic institutions, technology companies, and open-source communities frequently collaborating to advance the field. Open-source frameworks like TensorFlow, PyTorch, and HuggingFace’s Transformers have democratized AI development. However, geopolitical tensions and corporate competition sometimes limit knowledge sharing, leading to potential duplications of effort or nationalistic approaches to AI.
International collaborations—such as the Partnership on AI—attempt to establish guidelines for ethical AI and share best practices. These efforts aim to ensure that AI benefits humanity as a whole, rather than becoming a tool for dominance. Nonetheless, with nations viewing AI leadership as a strategic advantage, balancing cooperation and competition remains a tightrope walk.
18. The Complexity of Measuring AI Progress
Benchmarking is a crucial element for evaluating AI models and tracking progress, yet it can be misleading. Many benchmarks—like ImageNet for vision or GLUE for language—offer a snapshot of performance in contrived settings. Models that excel in these benchmarks can falter in real-world conditions or display unintended biases. Furthermore, once a benchmark becomes the focus of intense research, it can lead to “benchmark overfitting,” where incremental improvements are achieved without truly advancing AI’s general capabilities.
The call for more holistic evaluations, such as multi-task learning benchmarks and real-world challenge sets, has grown louder. Researchers are developing new benchmarks that test reasoning, generalization, and robustness, forcing AI systems to adapt to out-of-distribution inputs and more complex tasks.
19. The Cultural Impact of AI
AI’s influence extends into culture, art, music, and entertainment. Musicians use AI to generate new compositions, artists incorporate generative models to create novel visual pieces, and AI-driven content recommendation engines shape the cultural products people consume. Whether in blockbuster films highlighting dystopian AI or interactive exhibits in museums, AI has become a prevalent theme in modern storytelling.
This cultural interweaving offers a mirror, reflecting both our aspirations and anxieties about the future. While some view AI as an existential threat, others see it as a liberating tool that can expand human creativity and efficiency. The proliferation of AI-themed literature, games, and movies underscores its role as a defining narrative of our era.
20. Looking Ahead: Key AI Trends and Innovations
AI continues to push boundaries in multiple areas:
Multimodal Learning: Integrating text, images, audio, and sensor data within unified models.
TinyML: Deploying efficient ML models on resource-constrained devices for smart environments.
Neurosymbolic AI: Combining neural networks with symbolic reasoning for better interpretability.
Transfer Learning: Reusing knowledge from one domain to accelerate learning in another.
Meta-Learning: AI that learns how to learn, improving data efficiency.
Causal Inference: Enhancing AI’s ability to understand causal relationships, not just correlations.
Human-in-the-Loop Systems: Creating synergies between AI and human expertise for better outcomes.
These emerging directions signal that AI’s evolution is far from over. As data remains plentiful and computing resources continue to expand, new paradigms and breakthroughs are likely to surface in the coming years, shaping our world in ways yet unimagined.
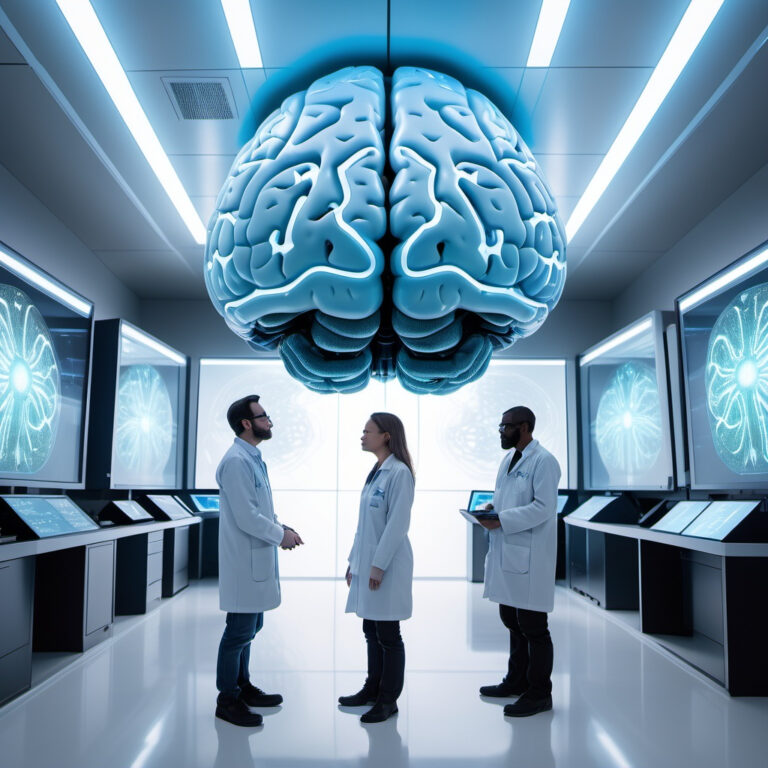
Conclusion
Artificial Intelligence stands at the core of a technological revolution that promises to redefine the contours of society, industry, and personal life. From its early symbolic logic origins to today’s powerful deep learning systems, AI has traversed an extraordinary journey, demonstrating achievements once believed impossible. It permeates every facet of modern life—healthcare, finance, entertainment, governance—and increasingly shapes critical decisions that affect global populations.
Yet, with immense power comes immense responsibility. Ethical considerations, data privacy, fairness, and the potential for harm through misuse are pressing concerns. Regulatory frameworks lag behind the breakneck pace of innovation, raising urgent questions about how best to manage AI’s impact on employment, resource consumption, and fundamental human rights. The same technology that can accelerate medical diagnoses can also enable intrusive surveillance; the same generative models that can create awe-inspiring art can also propagate deepfakes and misinformation.
As we stand at this crossroads, the guiding principle must be responsible innovation—leveraging AI’s capabilities for societal good while instituting checks and balances to mitigate risks. Interdisciplinary collaboration among engineers, policymakers, ethicists, and the public at large is crucial for ensuring that AI augments human potential without eroding the essential qualities that bind our societies together.
Looking to the future, AI’s scope will likely expand far beyond its current boundaries. Breakthroughs in quantum computing, neuromorphic hardware, and advanced algorithms could propel us into an era of exponential growth in AI capabilities. Whether these technological leaps lead to utopian outcomes or exacerbate societal divides depends largely on the choices we make today. By investing in transparent research, inclusive design, robust governance, and equitable access to AI benefits, humanity can guide the trajectory of AI to shape a future that is innovative, fair, and profoundly transformative.